Dynamic thresholds: some characteristics of dynamic monitoring
A threshold is a value used to change from one state to another in a check and dynamic monitoring is used to automatically adjust the thresholds of module states in an intelligent and predictive way. When the thresholds are defined by dynamic monitoring then we are talking about dynamic thresholds.
Depending on the threshold, a different state is defined; this way we will be able to find out the state of our check. Therefore, the state of the different checks will depend on these thresholds and from this information, we will be able to find out if the server, process, application or network element are working properly and we will also be able to find out if there is any anomaly or incidence so that we can launch the corresponding alerts in order to solve it.
Internally, at a low level, the operation of dynamic thresholds results in a collection of the values of a given period and a calculation of a mean and a standard deviation. It is necessary to establish a period so that the monitoring system can learn from the data collected, and also should be able to analyse which values are below or above the average and thus it will help us assess whether there are possible incidents in our IT infrastructure. If we give it a low learning time, such as five or ten minutes, the system will only have recent data so it is advisable to set a period of time of days or weeks so that more values are considered when performing these calculations to obtain the dynamic thresholds in a more solid way, through a greater number of values. Once the calculations have been carried out with the obtained data in order to obtain both the mean and the standard deviation, these are used to establish the corresponding dynamic thresholds in the modules automatically. These thresholds change, depending on the data that has been collected, the recalculation is performed, therefore, thresholds vary and adapt themselves to the new reality by applying the intelligence mentioned above about the data.
Under these premises, we obtain the following advantages in our monitoring tool when applying dynamic monitoring:
- It applies the thresholds automatically. The main feature is that once it has learned from the data collected in the defined time, thresholds are automatically applied in those modules we want, it is not necessary to define the thresholds manually. This task is automated and it is also customized according to the values of each module, which leads us to think about intelligent monitoring.
- It recalculates the thresholds. The system recalculates thresholds from time to time based on the information obtained, therefore these are updated with the values received.
- It provides flexibility when defining thresholds. Although dynamic monitoring is focused on automation, it is also possible to manually adjust a dynamic range in order to give greater flexibility to those automatically generated thresholds.
Once we have seen its operation and the advantages of monitoring our infrastructure using dynamic thresholds, we will put it into practice by looking at some examples.
Monitoring the web latency
We define a learning time of 7 days (a week) in the module:
Once the indicated configuration has been applied, the following thresholds have been defined:
This module will change its status to warning as soon as the latency is higher than 0.33 seconds and to critical state as soon as it is higher than 0.37 seconds. We represent these changes on the graph in order to see the action that will be carried out:
We can refine this more, since the threshold has been set high due to the peaks included in the monitoring. It is possible to reduce it by 20% so that alerts are triggered with a lower threshold. We will modify the values of the Dynamic Threshold Min. Field using a negative value so that the minimum thresholds fall. As there is no maximum value, since it will be considered critical from a certain time on, we do not have to modify the Dynamic Threshold Max:
After applying the changes, the thresholds have been recalculated, in this state:
Now, the graph looks something like this:
By applying the dynamic thresholds we have managed to define the thresholds, in addition to that, we have verified that apart from the calculation that is made to obtain the thresholds depending on the time, we can dig deeper and manage to adjust the changes of state even more according to our monitoring needs.
Monitoring the temperature in a CPD
The temperature in the CPD must be controlled and stable, so if you consult the monitoring graph, you will see the following information:
As you can see in the image, it is a linear graph that is always placed between the same values, it shouldn’t have sudden changes, which means that it shouldn’t rise or fall too much.
In this case we wanted to adjust the thresholds as much as possible, so we have manually defined a dynamic range with the following information:
We have enabled the “Dynamic Threshold Two Tailed” parameter to define thresholds both above and below. These are the dynamic thresholds generated:
These are shown in the graph:
This way, we are adjusting as much as possible to the temperature desired for the CPD, between 23 and 26 degrees. By looking at the graph, we can analyse that everything that is in the range between 23’10 and 26 is considered normal. Everything that goes beyond these thresholds will trigger the alerts.
The Pandora FMS monitoring software has dynamic monitoring
It establishes dynamic thresholds in the modules so it is not necessary to be aware of the definition of the thresholds for alerts and events. Let Pandora FMS do it for you.
You can find out more information about dynamic monitoring in Pandora FMS in the following link.
Our Pandora FMS team will be happy to answer your questions.
If you were interested in this article, you can also read: What is alert fatigue and its effect on IT monitoring?
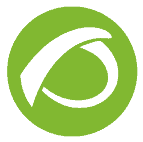
Pandora FMS’s editorial team is made up of a group of writers and IT professionals with one thing in common: their passion for computer system monitoring. Pandora FMS’s editorial team is made up of a group of writers and IT professionals with one thing in common: their passion for computer system monitoring.